Dendritic Spines, Memory, and Brain Preservation
Let me start this post with a disclaimer: I am not a trained neuroscientist. As VP and co-founder of the BPF, I enjoy following the scientific literature on neuroscience topics. I am instead a technology futurist, with six years of postbaccalaureate and graduate studies in biological sciences, computer sciences, and medicine at UCSD, and a master’s degree in futures studies from U. Houston. So my mind naturally goes to the longer-term implications of the advances we are seeing in these fields. And what advances they are! It’s quite an exciting and optimistic time to be alive.
This post will review some recent advances in the neurosciences, and what they may mean for human brain preservation in coming years. Speaking as a futurist, not a neuroscientist, some of this will be partly incorrect and speculative, but I hope you find it valuable nonetheless. I will try to indicate where the speculation starts, and please let me know when you disagree. It is only by explaining our models that others can help us correct them, and it is only by giving ourselves permission to tell plausible stories about the future, and to carefully criticize them, that our social foresight grows.
The most interesting neuroscience advance I saw in 2015 was in optogenetics. It was the establishment, labeling and optical erasure of a long-term synaptic memory in a mouse’s motor cortex. For the paper, see Hayashi-Takagi et al. (2015) in the References below. Akiko Hayashi-Takagi is a professor of medicine at the University of Tokyo. His team developed a transgenic mouse that expressed an optogenetic protein preinserted into the mouse’s dendritic spines, so they could see any of them change as the mouse learned a motor task. They then observed synaptic remodeling and dendritic enlargement in a very small subset of cortical neurons during the task. Then they used a fiber optic probe to shrink just a few of those enlarged dendrites, and the memory disappeared. This work supported longstanding theories by many neuroscientists that connectomics, and specifically the sizes, shapes and types of connectivity among dendritic spines, are the most fundamental way that long-term memories are stored in our brains. As Lu and Zuo at UC Berkeley speculate (see Yirka 2015), what Hayashi-Takagi’s team may seek to do next is learn how to selectively enlarge spines with their probe, so the mouse can acquire motor tasks without ever having been taught them, just by rewiring their dendritic circuits.
Dendritic spines are signal input channels that synapse onto neural cell bodies, where their firing is integrated in space and time so that the cell can decide when and how to generate an action potential. Generally, the larger the spine, the stronger the neural circuits and decision systems that it is a part of. An average of ten thousand spines of varying sizes typically synapse at various locations onto the cell bodies of each pyramidal neuron in mammalian hippocampus and cortex. As recent work by Bartol et al. (2015) at Salk argues, there may be as many as 26 different relevant sizes of these synapses, enabling a single human brain to store as much as a petabyte of information in its neural circuitry. A petabyte is a vast amount of information, but it is still small compared to the global web. Google, Amazon, Microsoft and Facebook together store about 1,200 petabytes between them. The web itself was estimated in 2009 to contain 500,000 petabytes. Keep in mind here that we are only referring to information stored in neural circuitry and synapses, which is presumably the highest order, most important information that we care about when we contemplate the prospect of preserving our selves, and in particular, our declarative and procedural memories.
Looking beyond our connectome, with its dendrites, synapses, and circuits, a vast amount of additional chemical and molecular information exists in any brain. Consider for example all the epigenetic and chemical information stored inside neurons, and in all their supporting cells, including glial cells, which are up to four times more plentiful than neurons in our cerebral cortex (you may have learned in Kandel’s Principles of Neural Science, that glia are “up to 50 times more plentiful than neurons”, but that no longer looks correct). All of this information is surely much greater than a petabyte per human being.
But how unique is this information to each of us, as individuals? How much would it hurt you if you lost that information in a future upload of your present self into a machine? Your glia are preserved in brain preservation, and all those chemicals and molecules as well. So if we need them in a future emulation, we’ll be able to upload those, too. But will we need to? Some folks in the neuroelectrodynamics community, who are trying to build a theory of mind based on the physics of electrical interaction in the brain, from the molecular level on up, might argue we will need a lot more than a unique connectome to capture our individual minds. Others, though, including several neuroelectrodynamicists, would argue that we may need such information in a generic human brain emulation, but not the unique versions of it in each of our brains. A lot of chemical and electrical information is constantly coursing through a brain, but most of it is there to keep the system alive. Only a small amount of this information supports our mind, and only a fraction of that information records our unique memories and personality. My current thinking, based on my read of the current literature, is almost all of our higher selves is likely to be stored in our connectome, including its unique morphology and receptor types and densities.
Here’s a thought experiment for you: If your connectome was preserved and uploaded into a generic brain emulation in a computer in the future, do you think you would still be mostly you? I would say yes, at present. I expect you would likely quickly grow into new personality going forward, as your new “substrate” would give you different learning abilities and proclivities. But I also expect your higher memories, and your memories of your past personality would be very well preserved, and you’d still feel very much like “you.” I don’t have a lot of evidence for this view at present. But as neuroscience and computational neuroscience continue to advance, and as we upload (emulate) simple animals neural circuits into computers in coming years, I bet we will gain a very good understanding on these issues long before the first human uploading occurs.
So let’s look closely now at this connectome, and at one particular aspect of them, dendritic spines, which are a key way to understand how our memories and personalities grow. Dendritic spines are typically excitatory at their synapses, using the neurotransmitter Glutamate, but a small number are inhibitory, using the neurotransmitter GABA instead. Add all this together and you’ve got tremendous stored neural complexity merely in the connectomics, the connectivity, shape and size and “sign” (usually excitatory, but occasionally inhibitory) of the “arbor” or tree-and-network structures of these neurons.
Again, a number of neuroscientists working to understand long-term potentiation (stable long-term changes in synaptic strength) and synaptic plasticity (the ways synapses change their strength) have long suspected that is primarily the sizes and connectivity patterns of these arbors that store our precious episodic memories (memories of self-related events and concepts). There’s another massive set of arbors that use inhibitory (GABAergic) rather than excitatory (Glutamatergic) synapses. These include spiny neurons in the basal ganglia and purkinje neurons in the cerebellum (our “little brain”). Just as pyramidal arbors store our episodic memories (autobiographical and conceptual learning), purkinje arbors are involved in filtering down a vast set of potential motor actions, and storing our procedural memories (motor learning). In both cases, the connectomics of intricate dendritic arbors may be the primary way that long-term stable learned information is stored. See this great Singularity Hub article, How the Brain Makes Memories, Jul 2015, by neuroscientist Shelly Fan of UCSF, describing how episodic memories are encoded (“incepted”) by single neurons, from single presentations of faces and places, and how these “engrams” interact with other neurons to add detail to a memory.
This arbor-based approach to understanding memory encoding, for all its value, may still be an oversimplification. For example, some neuroscientists now believe that parts of the neuron’s epigenome (in the cell nucleus) may be involved in learning and memory. Again, according to Ken, I’ve heard that the epigenome, according to the literature so far (and not by our own assessments), also appears well-preserved by both of the protocols presently competing for our prize. That is rather to be expected, as DNA, like the cytoskeleton of cells, is quite a stable macromolecule.
Most importantly however, the synapse, at the end of each of these spines has massive molecular complexity, and its essential features must be preserved too. One of the world’s leading synaptomics researchers, Stephen Smith (2014) estimates there are hundreds of protein species in every mammalian synapse, and he reminds us that our theories of how learning and memory work are still not deeply evidence-based. We may think we know most of the key neurotransmitters involved (Glutamate, GABA, 5-HT, Dopamine, acetylcholine, norepinephrine) and since the mid-1990’s we think we’ve now discovered several of the key plasticity proteins (such as Arc) that stably store our memory engrams, stitching them into the cytoskeleton of the synaptome. But we’re clearly still missing basic pieces of the story, and we haven’t yet built the big data sets and trained our machine learning systems to tell us, from the bottom up, what all the critical synaptic systems are.
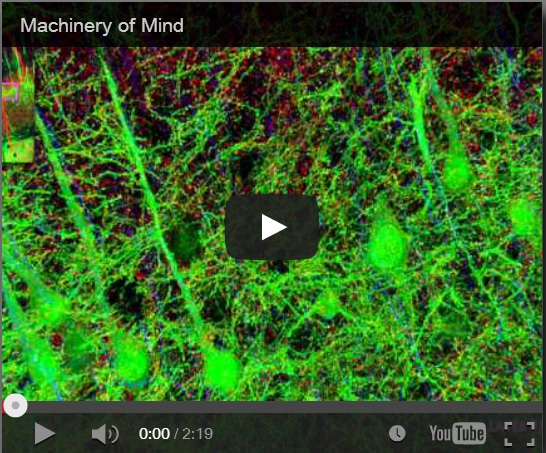
Synaptaesthesia (Machinery of Mind), Smith Lab, Stanford U (2012)
To address this problem, Smith’s team at Stanford pioneered a new proteomic imaging method, array tomography, that makes ultrathin slices of chemopreserved (plastic-embedded) neural tissue, transfers them to a coverslip, flourescent antibody stains and images them for a variety of key proteins and neurotransmitters, then uses software to reconstruct the most detailed 3D images of the synaptome and connectome that we have yet seen. Watch the Smith Lab’s mesmerizing brief video, Synaptaesthesia (Machinery of Mind) (picture right), for an array tomography-reconstructed section of one piece of a mouse’s whisker barrel, showing the six layers of somatosensory cortex and a bit of striatum below it. It’s an awe-inspiring demonstration of neural complexity. Smith estimates this is roughly 1/150 millionth of the human brain’s volume.
Clearly there’s enough complexity in these arbors and their synapses to represent a lifetime of human memory and identity. Fortunately, tools like array tomography show us that we now have the ability to capture that complexity at the time of death, using either chemopreservation (“plastination”) or aldehyde-stabilized cryopreservation, and store it for an arbitrary number of years until we can figure out how it works. That’s a very exciting realization. This new ability, when reliably scaled to whole brains, and combined with the rapid progress we are seeing in automation and computational neuroscience, makes it a reasonable bet that we’ll be able to affordably “upload” all this information into a much faster, more flexible, and efficient virtual form, perhaps even within this century. As deep learning continues to accelerate in its abilities (Google DeepMind’s AlphaGo will go up against the top Go player in the world in March) more of the doubters on this particular point may be convinced. Fortunately, we’ll have plenty of years before uploading arrives to see if machine learning continues to improve much faster, more flexibly, and more efficiently than human learning has over evolutionary history. Consider this simple observation: Biological neurons think and learn at roughly 100 miles per hour, using chemical action potentials, and artificial neural networks “think”, or learn from rapidly growing electronic data, at the speed of light, using electrons. The latter system is vastly more “densified and dematerialized” in its critical computational processes, as I discuss in my forthcoming book, The Foresight Guide (2016).
The big win, from my perspective (more speculation here) comes when people start to realize that our information technologies, including both the global web and our personal sims–conversational personal software agents, arriving as soon as the end of this decade, that model our interests and help us advance them–are rapidly becoming a natural extension of our biological selves. We human beings are becoming continually growing and learning “informational entities,” a mix of both our biological and technological networks. This fortuitous development may happen in every corner of the universe when biological intelligence develops information technology, so this fusion may be less a matter of human creativity than human discovery of natural efficiencies in our universe’s physics of computation. What mix we are of the two (biology and technology) seems much less important than that our important patterns are both preserved from degradation and always susceptible to feedback, renewal, and improvement.
We must remember that neural networks, whether biological or artificial, eventually become overtrained and brittle. The only way out of that trap is rejuvenation and retraining. We are a long way from figuring out how to do that with human biology, but we are already learning how to do that renewal with our deep learning machines. Therefore, the more technological we become, the more every one of us can reunderstand ourselves, at at least the technological portions of ourselves, as lifelong learners, as perpetual children, experimenters, and investigators. I’ll have more to say on that topic as well in The Foresight Guide. I believe that our emerging personal digital learning abilities will make us far less inflexible, dogmatic and judgmental of others. When it isn’t so very hard to change our views, every position becomes more lightly held, able to be improved as new theories and data come in.
I also believe (more speculation) that there will be many more big simplifications we’ll discover in the neuroscience of learning and memory, both in the top-down, theory driven way that has dominated the field so far, and in the bottom-up, machine-learning, data-driven way that Smith advocates. These simplifications will tell us just what features of all this synaptic and connectomic diversity are key to individual memory and identity, allowing us to ignore a much larger set of cellular and physiological processes that are there to keep our neurons alive. I think it is a special subset of our neural complexity that stores, in a stable way, the higher information we care about. That’s clearly what neuroscience has been arguing so far. You and I can understand each other in conversation not because of the diversity in our synapses, but because of some deep developmental commonalities to our neural arbors. Figure out those commonalities, our “baseline brain”, and we’ll also get a much better picture of the systems that store our precious individual differences in thinking, emotion, and personality on top of that brain architecture and function we all share.
There is also a very old idea in the neuroscience of learning and memory that short-term memories, in both hippocampus and cortex, are mediated by lots of short-term protein changes in the synapse (recall Smith’s synaptic diversity), but long-term memories require either new synapse formation, synapse enlargement, or other obvious morphological connectomics changes. If that simplification turns to be true, then the first benefit that we may see in brain preservation technologies will be the ability to know that our long-term memories, at least, can be read and uploaded into virtual form in the forseeable future, as morphology scanning and reconstruction is a particularly tractable computational task. We are already doing morphology scanning and selective protein tagging using human-supervised machine learning approaches, with very small portions of mammalian brains today, as the movie above shows. Fully automated and unsupervised machine learning classification approaches are clearly coming in the future as well. So as we will now discuss, long-term memory retrieval from model organisms may turn out to be the first proof of principle of the value of the brain preservation choice.
Some scientists are already offering some elegantly simple morphological memory hypotheses for long-term memory. The distinguished neuroscientists Bourne and Harris (2007) tell us that in adult human hippocampus and cortex, roughly 65% of our spines are ‘thin’, about 25% are ‘mushroom’ spines, and the remaining 10% are stubby, branched, or another variety of ‘immature’ forms. See the picture at left for the different shapes. In their paper, restating them in layman’s terms, Bourne and Harris propose that thin spines are what we do our thinking with, interpreting our sensory data and relating it to our memories and motor outputs, and mushroom spines are where we store our stable long-term memories. Many of these mushroom spines are strongly attached to their postsynaptic cell body via anchoring proteins called cadherins (cell-to-cell adhesion proteins), which presumably makes a morphological memory so stable that it will persist for a lifetime, through all the normal cellular and extracellular biochemical and osmotic changes that constantly occur in living cells. A special kind of supramolecular assembly called a cadherin-catenin complex has been implicated in the signal transmission process that controls synaptic plasticity. Again, to oversimplify a bit, if the Bourne and Harris model proves true, about one quarter of the circuitry of the human brain is dedicated to memory storage, and the rest is dedicated to thinking, both about our outside world, and our own memories. We may be 75% thinking, and 25% memory machines. Pretty neat, huh?
Is the architecture of mushroom dendritic spines in fact the “hard drive” of our brains? Are all of our spines and their synapses, in cortex, thalamus, and a few other key brain areas, what we really need to most carefully preserve, in brain preservation protocols, in order to later read out long-term memory? Perhaps it’s too early to say for certain, but I’m now a big fan of them. A good book on spines, which explores how they form neural circuits and networks to do memory storage, computation and pattern recognition, is Rafael Yuste’s Dendritic Spines (2010).
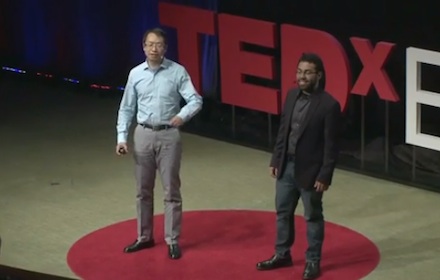
A mouse. A laser beam. A manipulated memory. Liu and Ramirez, TEDxBoston 2013 (TED.com, 15 min)
Looking back two years ago now, you may also have heard about the Liu and Ramirez (Dec 2013) paper, where a mouse’s memory was erased and then “incepted” (introduced) back into its hippocampus, again using optogenetics with a very small population of neurons. These scholars did a great TED talk in 2013, which generated 1 million views (it should have generated 20 million!). See also this nice writeup of their work by Noonan (2014). Via optical probes, they were even able to alter connections from the hippocampus to the amygdala, which modulates emotions, and turn a mouse’s fear memory into positive memory, in relation to a particular place. Unfortunately Liu, just 37, tragically died of a heart attack last year, but Ramirez continues this amazing work.
While the Liu and Ramirez work is bold and ingenious, I also found it difficult to interpret, as our hippocampus apparently stores both short-term memories (the last few days) and pointers to our long-term memories (stored in cortex), and it does so in a way that doesn’t always involve spine enlargement and making new synapses, but is often just restricted to molecular changes to existing synapses. So hippocampal arbors may need to be more multifunctional than cortical arbors, and may work a little differently. The hippocampus is also the only place in our brain where neural stem cells are constantly budding, to replace damaged neurons and their dendritic trees, presumably because they are so heavily used for short-term memory storage and “pointing” to other places in our brains. For more on the hippocampus, and why preserving it, while certainly ideal, may actually not be as important as our cortex and the rest of our brain, see my 2012 post, Preserving the Self for Later Emulation: What Brain Features Do We Need? So for me, it took the Hayashi-Takagi experiment to finally become optimistic about how simple and morphological long-term memory storage in human cortex may turn out to be.
According to Ken Hayworth, President of the BPF, as far as we can tell today, dendritic size, shape and connectivity information appears well-preserved in the protocols being used by both of the current competitors for the Brain Preservation Prize. Of course, in addition to morphology, some additional amount of molecular, and receptor information in the synapses (remember Arc and those cadherin-catenin complexes, for example) and perhaps some epigenetic information in the cell nucleus, may be necessary to reconstruct memories. Whatever information that turns out to be, we will have to ensure it is protected.
One way we’ll know we have protocols that are up to the task is when neuroscientists have successfully preserved, scanned (“uploaded”), and retrieved long-term memories from well-studied neural circuits in model organisms. I think this “memory retrieval” challenge is one of the frontiers of computational neuroscience. Neuroscientists are already using tools like FIB-SEM, and semiautomated multibeam scanning systems, to “upload” very small animal brains, like nematodes, zebrafish, and portions of fly brains. Ziess, one of the leading manufacturers of FIB-SEM machines, is now making a 61-beam machine that can parallelize connectomic scanning, and even more parallelized and automated scanning systems will emerge as connectomics scales. These brains and brain sections are on the order of the size of a tip of a pencil. We don’t yet understand how long-term memories are stored in these brains, at least not episodic and conceptual memories. So computational neuroscientists don’t yet have the knowledge needed to read these uploaded circuits. But all this recent progress in neuroscience and related progress in deep learning in computer science (a topic we will leave to another time) give me hope that we will get that knowledge, and episodic memory retrieval from a well-studied set of circuits in a scanned animal brain will be demonstrated in a computer within this decade (more speculation).
Once we can successfully predict, by looking either at live or uploaded versions of neural arbors and their synapses, how a complex animal has been trained in a spatial or conceptual task, in blinded experiments, given a large variety of initial training options, we’ll know we’ve cracked the long-term memory problem. An impressive version of memory retrieval from scanned brains might happen first in relation to the animals’ spatial model of the world, a complex neural representation system that we’ve recently begun to understand. See Wikipedia’s pages on hippocampal place and grid cells for more. The team that discovered grid cells, how animals use special networks of neurons to represent their position in space, won the 2014 Nobel prize in Physiology and Medicine.
When we can retrieve one of these high-level memories out of preserved and scanned brains, we’ll know that brain preservation protects something very valuable, our long-term memories, and the ways we tend to think about them. Knowing whether these protocols will preserve other things, like our unique personality and consciousness, whether they can bring “us” back, I think we will eventually get there, but more slowly . Consciousness, for example, is still not well understood in neuroscience. Though neuroscientists offer promising materialist models such as neural synchrony (see Buzsaki 2011), no model is yet validated or widely agreed upon in the neuroscience community, and the mechanisms of consciousness, which may include little-understood phenomena like ephaptic coupling, are still unclear.
So for me at least, memory preservation is the first proof of value for these technologies. In addition to the great value of brain preservation work for neuroscience, medical science, and computer science, it seems likely to also have great personal value, for those who think their life’s memories are worth preserving and giving to future loved ones, to science, or to society in virtual form. If I think a protocol is likely to preserve my long-term memories and thinking patterns at the time of my death, I will be very interested in it, both for myself and my loved ones, if it can be done both affordably and sustainably. If it preserves anything beyond that, if “I” feel like I have come back in that future world, that will be a great bonus, but not necessary to establish its basic value.
As further progress occurs in the neuroscience of learning and memory, as well as in the computational neuroscience that uses neural network and other approaches to store increasingly biologically-inspired “memories” in computers, the BPF will continue to investigate whether the best protocols available to neuroscientists, computational scientists and medical professionals are fully up to the task of preserving our own memories and identity, for all who might desire to have the preservation choice available to them at the time of their biological death. Thanks for reading.
References
Bartol et al. (2015) Nanoconnectomic upper bound on the variability of synaptic plasticity (Full Text). eLife 2015 Nov 30;4:e10778.
Bourne and Harris (2007) “Do thin spines learn to be mushroom spines that remember? (PDF), Curr. Opin. Neurobio 2007, 17:1-6.
Buzsaki, Gyorgy (2011) Rhythms of the Brain, Oxford U. Press.
Hayashi-Takagi et al. (2015) Labeling and optical erasure of synaptic memory traces in the motor cortex (Abs). Nature 2015 Sep 17;525(7569):333-8.
Liu et al. (2013) Inception of a false memory by optogenetic manipulation of a hippocampal memory engram (Full Text). Phil. Trans. Royal Soc. B 2013 Dec 2;369:20130142.
Noonan, David (2014) Meet the Two Scientists Who Implanted a False Memory Into a Mouse, Smithsonian, Nov 2014.
Ramirez and Liu (2013) A mouse. A laser beam. A manipulated memory. TED.com, TEDx Boston, Jun 2013 (15 mins)
Smart, John (2012) Preserving the Self for Later Emulation: What Brain Features Do We Need?, EverSmarterWorld.com Sep 24, 2012.
Smith, Stephen (2012) The Synaptome Meets the Connectome: Fathoming the Deep Diversity of CNS Synapses, Talk, SCI Institute, 6 Mar 2012 (YouTube, 69 min)
Smith Lab (2012) Synaptaethesia, Stanford U. School of Medicine (YouTube, 5 min)
Yirka, Bob (2015) Researchers erase memories in mice with a beam of light. MedicalExpress.com Sep 11, 2015.
Yuste, Rafael (2010) Dendritic Spines, MIT Press.